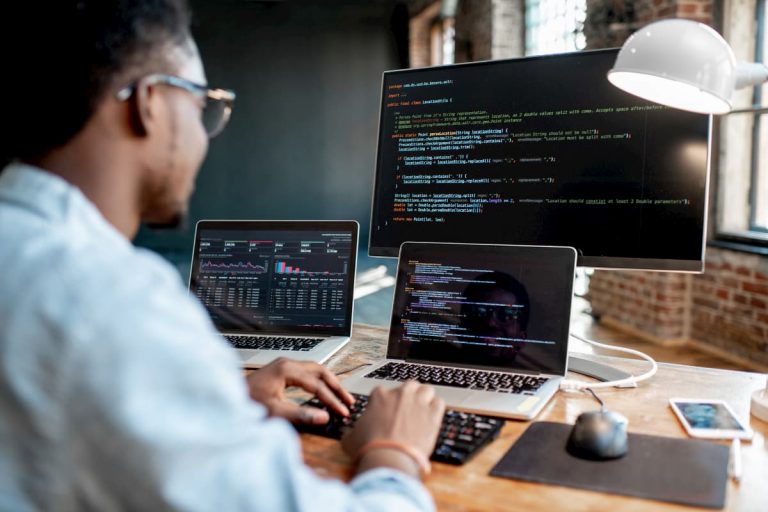
In the realm of Artificial Intelligence (AI), data serves as the foundational layer upon which all intelligent systems are built. The data layer encompasses the vast, intricate networks of information that AI algorithms use to learn, adapt, and ultimately perform tasks that would otherwise require human intelligence. As we delve into the data layer, we navigate a complex landscape that is both fascinating and challenging.
The data layer is not just about the quantity of data but also about its quality and structure. High-quality, well-structured data can significantly enhance the performance of AI systems. It’s akin to providing a clear, well-drawn map to a traveler in an unknown city; it makes navigation easier and more efficient. Conversely, poor-quality data can lead to AI systems making inaccurate predictions or decisions, much like a traveler relying on a faulty map.
One of the critical aspects of navigating the data layer is understanding the statistics that underpin AI models. Statistics provide the framework for making sense of data, from identifying patterns to making predictions. For decision-makers and professionals looking to harness the power of AI, developing a nuanced understanding of data is crucial. Courses like “Understanding Data: Navigating Statistics, Science, and AI” offered by Michigan Online and Coursera’s specialization on the same topic equip individuals with the essential skills to critically engage with data and AI narratives.
Tekedia Mini-MBA edition 16 (Feb 10 – May 3, 2025) opens registrations; register today for early bird discounts.
Tekedia AI in Business Masterclass opens registrations here.
Join Tekedia Capital Syndicate and invest in Africa’s finest startups here.
Another layer to consider is the intersection of machine learning—a subset of AI—and data analytics. Machine learning algorithms learn from data without being explicitly programmed, and data analytics focuses on processing and interpreting vast datasets. The synergy between these two fields is powerful, enhancing analytical processes and empowering businesses to navigate complexities with agility.
Managing AI data is a complex task that involves various challenges, which can significantly impact the success of AI projects. Here are some common hurdles encountered in the process:
Data Quality and Preparation: Ensuring high-quality, clean data is available for training AI models is a significant challenge. Data often requires extensive cleaning and preprocessing to be usable, which can be time-consuming and resource intensive.
Data Integration: Combining data from diverse sources to create a unified, coherent dataset is another common obstacle. This integration is crucial for the AI to have a comprehensive understanding and make accurate predictions.
Talent Shortage: There is a notable scarcity of skilled professionals who can manage and interpret AI data effectively. The lack of expertise can hinder the development and implementation of AI initiatives.
Moreover, the rise of AI has brought about discussions on privacy and data protection. Organizations deploying AI tools must consider how their systems collect, process, and disseminate personal data. Navigating the data layer, therefore, also involves understanding the ethical implications and ensuring that AI systems respect privacy and data protection standards.
Navigating the data layer of AI is a multifaceted endeavor that requires a critical understanding of data, statistics, and the ethical considerations surrounding AI. As AI continues to evolve, the data layer will only grow in complexity and significance, making it an ever-important field for study and exploration. For those looking to make informed decisions in an increasingly data-driven world, the journey through the data layer of AI is both a challenge and an opportunity for growth and innovation.