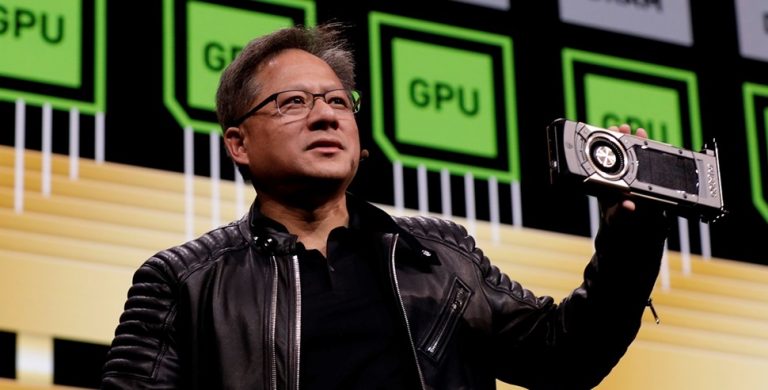
In a recent interview at the Hong Kong University of Science and Technology, Nvidia CEO Jensen Huang shared candid insights into the state of artificial intelligence (AI) and Nvidia’s contributions to its development.
Huang discussed the persistent issue of AI hallucinations, explained key advancements in AI development, and reflected on his career journey, including personal anecdotes about how he set high expectations for himself from an early age.
AI hallucinations, a notable challenge in artificial intelligence, occur when models like chatbots or generative AI systems produce outputs that sound plausible but are factually incorrect or entirely fabricated. It often stems from incomplete or biased training data, poor model architecture, and overfitting or underfitting during development.
Tekedia Mini-MBA edition 16 (Feb 10 – May 3, 2025) opens registrations; register today for early bird discounts.
Tekedia AI in Business Masterclass opens registrations here.
Join Tekedia Capital Syndicate and invest in Africa’s finest startups here.
These errors, distinct from AI biases or simple mistakes, have real-world implications across various domains.
Addressing the phenomenon—Huang was forthright about the challenges involved. He stated that resolving this issue would likely take several years, despite the progress in AI technologies.
“Today, the answers that we have are the best that we can provide,” Huang explained. However, he emphasized that current systems require users to evaluate whether an AI-generated answer is sensible or fabricated. He noted, “We need to get to a point where the answer that you get, you largely trust,” but acknowledged this milestone is still years away.
During the interview, Huang outlined three critical areas of AI development. The first stage is pre-training, which he likened to a college education, where the AI ingests vast datasets to build foundational knowledge. Next is post-training, which involves refining the AI’s capabilities in specialized tasks through techniques like reinforcement learning, synthetic data generation, and multipath learning.
The final stage, Test Time Scaling, represents what Huang described as “thinking.” Here, AI systems break down problems step by step, iterating and simulating outcomes to enhance solution quality.
“Today, the answers that we have are the best that we can, but we need to get to a point where the answer that you get is not the best that we can provide, and somewhat you still have to decide whether is this hallucinated or not hallucinated, does this make sense, is it sensible or not sensible? We have to get to a point where the answer that you get, you largely trust.”
He also added, “I think that we’re several years away from being able to do that, and in the meantime, we have to keep increasing our computation.”
Despite the challenges, Huang expressed optimism about the role Nvidia has played in AI’s evolution. He highlighted that Nvidia’s groundbreaking work has drastically reduced the cost of computation by a factor of a million. This, according to Huang, has fundamentally altered researchers’ habits, enabling exhaustive data analysis without hesitation.
“What Nvidia has contributed is that we’ve taken the marginal cost of computing and reduced it by a million times.” He said, “When the cost of something reduces by a million times, your habits fundamentally change…that is the single greatest contribution Nvidia ever made, that we made it so that using a machine to learn exhaustively an enormous amount of data is something that researchers wouldn’t even think twice to do. That’s why machine learning has taken off.”
The conversation also touched on Nvidia’s skyrocketing demand and the increasing need for computing power in AI. Huang refrained from predicting whether AI computing demands would continue to multiply at the same exponential rate over the next decade but acknowledged the trend’s transformative implications.
A host at the event highlighted that the demand for AI computing has grown fourfold annually, translating into a million-fold increase over a decade, a trajectory that has underpinned Nvidia’s meteoric stock performance.
Responding to critiques about the high price of Nvidia’s AI GPUs, Huang defended the costs by emphasizing the massive discount Nvidia has effectively offered over the past decade.
“It’d be a million times more expensive if Nvidia didn’t exist,” he quipped. “I gave you a million times discount in the last 10 years. It’s practically free!”
On a personal note, Huang recounted how he wooed his wife by promising he would become a CEO by the age of 30—a goal he achieved. The anecdote added a lighter, more human touch to a discussion largely focused on the technical and economic dimensions of AI development.
Strategies like using diverse datasets, refining training methods, and employing cross-verification tools like Nvidia’s NeMo Guardrails have been recommended to mitigate AI hallucination risks?.