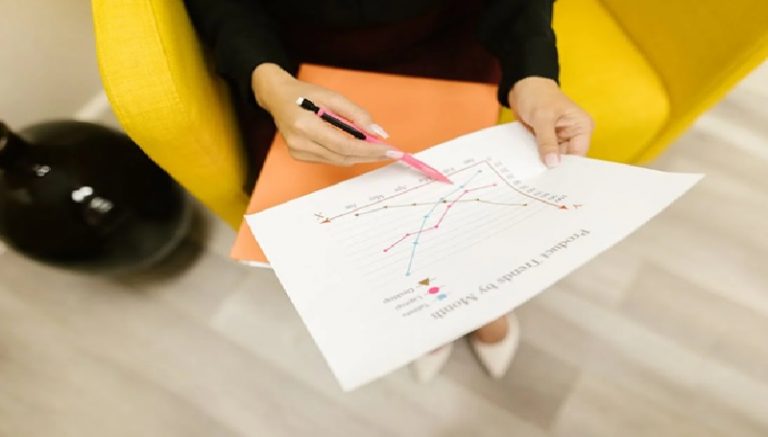
The rapid growth of digital payments has brought unprecedented convenience and opened new doors for fraudsters. Cybercriminals are evolving their tactics, making traditional security measures increasingly ineffective. As financial transactions become more seamless, so do the risks associated with fraudulent activities. Financial institutions and fintech companies are turning to artificial intelligence (AI) for a more sophisticated approach to combat this. AI-driven solutions redefine payment fraud detection, providing real-time analysis and predictive capabilities beyond conventional methods. By leveraging AI, businesses can identify fraudulent activities faster, reduce financial losses, and protect consumers from cyber threats.
The Role of AI in Fraud Prevention
The analysis capabilities of AI revolutionized fraud prevention when it came to processing unlimited data volumes beyond the speed of human analysts. AI surpasses traditional rule-based systems because it adopts changes in fraudulent patterns that mere detection parameters cannot tackle. Machine learning models display an automatic learning ability from new fraud attempts, allowing them to improve accuracy while preventing false positives.
Tested algorithms alongside deep learning approaches find hidden transaction activities through their ability to detect behavioral patterns. Computer systems track spending patterns, device behavioral data, and location data to define normal customer activities. The AI system monitors unusual banking patterns, including transactions from unknown locations, and attempted enormous withdrawals, which it then alerts for human review. The absolute real-time observation enables financial entities to prevent fraudulent transactions before they cause damage.
AI actively participates in predictive analytics operations along with its real-time detection functions. AI uses past fraud data analysis to project potential dangers and then provides organizations with intervention recommendations. This process enables organizations to enhance their security structure, thereby detecting defects that could otherwise become fraudster entry points. AI-driven detection systems undergo parallel development with fraud techniques to maintain an advantage over criminals without needing to respond after fraud occurs.
AI’s Impact on Reducing False Positives
The most significant problem in fraud detection occurs when organizations need to balance protecting users and providing them with a positive experience. Current fraud detection systems based on traditional methods produce an excessive number of incorrect alerts that mark valid transactions as suspicious. These alerts negatively affect Customers’ experience, resulting in payment declines, security check interruptions, and potential business losses. AI solves this challenge by improving accuracy and refining the risk assessment model.
Through machine learning, AI separates real anomalies from actual fraud attempts. Advanced models process multiple attributes, including transaction pattern counts, historical transaction patterns, and money flow dynamics to identify real security risks. AI-based fraud detection equipment enhances transaction security by reducing false alarms, enabling customers to complete transactions seamlessly without facing fraud attempts.
Fractionated payment fraud tools that use natural language processing (NLP) look through customer interactions, support requests, and emails for red flags of possible payment fraud. AI systems help when fraudsters pretend to be authentic users because they assist customer service representatives in avoiding unauthorized transaction approval. AI uses fraud-related linguistic patterns to safeguard financial systems without disturbing valid customer support activities.
The Future of AI in Payment Security
AI continues to develop, and its capabilities in detecting fraud will grow in forthcoming years. Deep learning and federated learning capabilities will enhance AI systems to become more innovative while operating autonomously. The AI model training method federated learning lets users train their models through distributed data while keeping their confidential details protected. Financial institutions can work together on fraud detection while safeguarding customer privacy through this system, which builds an enhanced networked protection against cyber attacks.
AI demonstrates promising advancements through its combination with blockchain technology. Blockchain operates outside of a centralized system, which adds an obstacle for manipulations from fraudsters in their attempt to modify transaction records. Simultaneously AI systems track blockchain actions to spot irregular behavior patterns. A combined blockchain-predictive AI framework demonstrates significant promise for digital security since it unifies blockchain transparency with AI analytic abilities.
The upcoming role of artificial intelligence appears decisive for regulatory compliance purposes in the industry. Under new regulations, implementing advanced fraud prevention measures has become mandatory for financial institutions, so AI automation delivers an effective solution. Through monitoring enabled by AI, companies can guarantee their compliance with both anti-money laundering (AML) and know-your-customer (KYC) regulations, which protects them from financial penalties and maintains their good reputation.
Conclusion
The fintech industry benefits from AI technology, leading to drastic changes in payment fraud detection methods. Machine learning, deep learning analytics, and predictive analysis allow AI-driven fraud detection systems to operate with high precision while delivering instant responses using minimal false detection rates. AI remains essential for fighting cybercrime since fraudulent techniques continue to adapt in their methods. Financial institutions that use AI-driven security solutions protect their customer base and develop their own resistance against new threats in the process. AI is the prominent payment security technology because it predicts and stops fraud attempts during their initial stages.