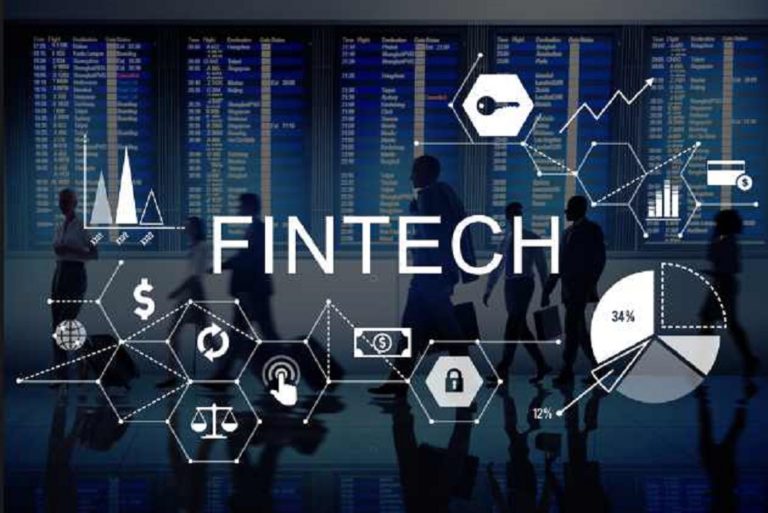
In 2006, Netflix created an open competition that would pay the winning data scientist a $1m prize. The task was to build a model that could outperform Netflix’s recommendation engine by 10%.
The winning team used an advanced machine learning technique known as “random forest,” which is now commonly used to optimize for predictive power. This incredible boost in predictive power, however, came at the cost of interpretability. While the winning algorithm best predicts how the next thousand users will rate various films, it cannot directly explain why Henry from Kansas enjoys Space Jam.
When it comes to fintech, “black box” A.I. is often impractical. Traditional players such as banks or insurance carriers may feel uneasy, and imagine telling a regulator you denied someone a loan because “the model said so.”
Tekedia Mini-MBA edition 16 (Feb 10 – May 3, 2025) opens registrations; register today for early bird discounts.
Tekedia AI in Business Masterclass opens registrations here.
Join Tekedia Capital Syndicate and invest in Africa’s finest startups here.
As a result, the most successful machine learning applications in fintech may be those that leverage unique alternative datasets, rather than the cutting edge of artificial intelligence.
Financial technology, also known as fintech, is an industry composed of companies that use new technology and innovation with available resources in order to compete in the marketplace of traditional financial institutions and intermediaries in the delivery of financial services. Financial technology companies consist of both startups and established financial and technology companies trying to replace or enhance the usage of financial services of incumbent companies.
That is why fintech will lag in pioneering AI because explaining to regulators that all decision making has been moved to models will be challenging in the foreseeable future. Regulators expect financial institutions to be making decisions by humans with machines supporting.
So even if you use machines, you need to have a clear understanding of the result so that humans can explain same to regulators. The implication is that if the models deliver great results and you cannot explain same. you cannot deploy them. That means your AI cannot be pioneering to the extent that humans cannot understand what is going on.
Fintech AI will lag voice, search, and other related areas which are not as heavily regulated as finance.